Introduction to Artificial Intelligence
Artificial Intelligence (AI) signifies the simulation of human intelligence in machines programmed to think and learn. By enabling machines to mimic human cognition, AI aims to simplify and enhance numerous tasks across various domains.
The importance of AI in today’s technological landscape is profound as it drives innovations, optimizes processes, and improves decision-making frameworks in industries ranging from healthcare to finance.
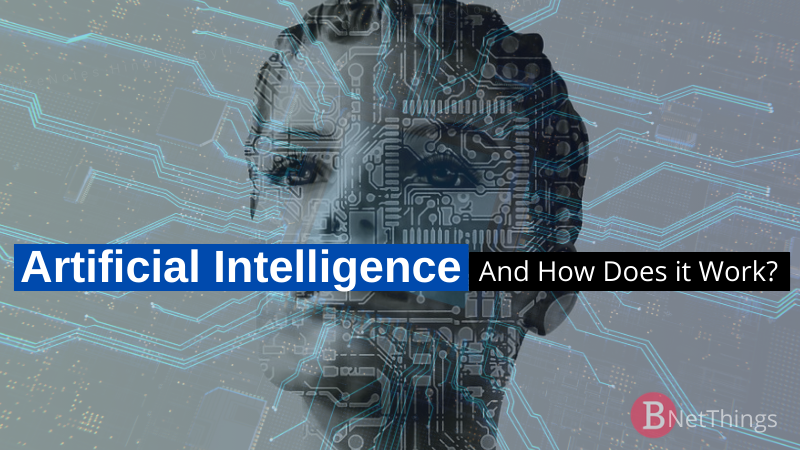
Historically, AI dates back to the ancient Greeks, who speculated about mechanical men, and to the medieval concept of automata. However, the modern foundation of AI started in the mid-20th century.
The term “Artificial Intelligence” was officially coined by John McCarthy in 1956 during the Dartmouth Conference, where AI was envisioned as the creation of intelligent agents capable of performing tasks that would typically require human intelligence.
AI has since evolved through various phases, from rule-based systems of the 1980s to the machine learning boom driven by big data and high computational power in the 2000s.
Present advancements focus on deep learning, a subset of machine learning, which has led to significant breakthroughs in areas such as natural language processing, image recognition, and autonomous systems.
AI can be categorized into different types based on its capabilities and functionalities. Narrow AI, also known as weak AI, is designed to perform specific tasks and operates within a predefined range of functions. Examples include virtual assistants like Siri and Alexa.
The concept of superintelligent AI refers to AI systems that surpass human intelligence and capabilities in every domain, which remains a theoretical possibility at present.
Understanding these categories helps to appreciate the broad spectrum of AI technologies and their potential. As AI continues to advance and integrate more deeply into our daily lives, its study and application become increasingly vital, underscoring the incredible potential it holds for the future.
Key Components and Technologies Behind AI
Artificial Intelligence (AI) encompasses a broad array of technologies and methodologies, each serving a crucial role in its development and functionality. At the heart of AI lies machine learning, a subset where systems learn from data, identifying patterns to make informed decisions.
In machine learning, algorithms—such as decision trees, support vector machines, and neural networks—process vast datasets, which are pivotal for training models to recognize and predict outcomes. This ability to learn from data is foundational to many AI applications, from recommendation systems to fraud detection.
Deep learning, a further specialization within machine learning, utilizes layered neural networks, sometimes called artificial neural networks, to emulate human brain activity. These networks excel at processing unstructured data, such as images and speech. Deep learning’s immense power is showcased in applications like autonomous driving and complex game playing where the model’s complexity and training depth directly impact performance and accuracy.
Natural Language Processing (NLP) is another cornerstone technology, enabling machines to understand, interpret, and generate human language. From chatbots providing customer service to sophisticated translators breaking language barriers, NLP leverages algorithms to parse text, sentiment analysis, and syntactic structures. Advances in contextual understanding now allow AI to engage in more nuanced and meaningful conversations, substantially elevating the user experience.
Computer Vision involves the interpretation of visual data by machines, replicating human vision capability. This tech is instrumental in various sectors like healthcare for diagnosing medical images, surveillance systems enhancing security, and e-commerce for improving product searches using visual cues. With the aid of convolutional neural networks, computer vision can identify and classify objects within images or videos, pushing the envelope in what AI can visually analyze and process.
In the realm of robotics, AI delivers sensory processing, decision-making, and autonomous action. Robotics integrates components of machine learning, computer vision, and NLP, creating systems that can perform tasks ranging from industrial automation to personal assistance. The fusion of these technologies orchestrates robots that interact with human environments more intuitively and efficiently.
The backbone of AI systems comprises advanced algorithms, extensive datasets, and substantial computational power. High-performance computing resources enable the training of complex models, while the availability of large-scale data ensures that these models can learn effectively.
Together, these elements form the building blocks of AI, driving innovation and paving the way for sophisticated and intelligent applications across diverse domains.
How AI Works: Core Processes and Methodologies
Artificial Intelligence (AI) operates through a set of foundational processes and methodologies that empower it to learn from data, make decisions, and improve itself over time. Key among these methodologies are the learning models classified as supervised learning, unsupervised learning, and reinforcement learning.
Each of these models has unique characteristics and applications, contributing to AI’s versatility and effectiveness in handling diverse tasks.
In supervised learning, AI systems are trained using labeled data, meaning the input data comes with corresponding output labels. This form of learning is akin to teaching a child with the help of a guide.
The AI system learns from these examples, identifying patterns and relationships, allowing it to predict outcomes for new, unseen data. This approach is widely used in classification tasks like image recognition and spam detection.
Conversely, unsupervised learning deals with unlabeled data. Here, the system attempts to discern patterns and structures from the input data without explicit guidance.
This method is particularly useful for clustering and association tasks, such as customer segmentation in marketing and anomaly detection in network security.
By identifying hidden patterns in the data, unsupervised learning provides insights and categorizations that might not be immediately apparent.
Reinforcement learning is slightly different, focusing on training AI agents through a system of rewards and punishments. The AI learns optimal behaviors by interacting with an environment and receiving feedback based on its actions. Over time, it adjusts its strategies to maximize cumulative rewards.
This methodology has proven especially successful in fields such as robotics, game theory, and self-driving cars. Central to the functioning of these learning models are neural networks and optimization techniques. Neural networks, inspired by the human brain’s structure, consist of interconnected layers of nodes that process data and recognize complex patterns.
Optimization techniques, such as gradient descent, are employed to fine-tune the neural network parameters, ensuring the model’s output aligns as closely as possible with the desired outcomes.
An often-overlooked yet critical aspect of making AI work effectively is data preprocessing and feature engineering. Data preprocessing involves cleaning and transforming raw data into a format suitable for training, while feature engineering focuses on selecting and creating meaningful input features that enhance the model’s performance.
Together, these steps ensure that the AI system is built on robust, high-quality data, facilitating more accurate and reliable outcomes.
Applications and Future of AI
Artificial Intelligence (AI) is rapidly transforming a wide range of industries by facilitating more efficient, accurate, and innovative approaches to various challenges.
In the healthcare sector, AI is revolutionizing patient care through predictive analytics, automated diagnostics, and personalized treatment plans. For instance, AI algorithms are now capable of accurately detecting diseases like cancer and diabetic retinopathy from medical imaging, potentially saving countless lives by enabling early intervention.
In the financial industry, AI enhances decision-making processes by assessing risks, detecting fraudulent activities, and optimizing trading strategies. Systems utilizing AI can analyze vast datasets to identify patterns and trends, providing actionable insights that drive business growth. Automated financial advisors, or robo-advisors, are an example of how AI simplifies personal finance management by providing customized investment advice and portfolio management.
The automotive sector is witnessing significant shifts with AI-powered advancements in autonomous driving and smart vehicle technologies. Companies such as Tesla and Waymo are at the forefront of developing self-driving cars, which incorporate AI for navigation, obstacle detection, and decision-making on the road. These innovations promise to enhance road safety and revolutionize transportation.
AI is also making waves in the entertainment industry through applications like recommendation systems, which personalize user experiences on platforms like Netflix and Spotify. Additionally, AI-driven content creation tools are being used to generate music, art, and even screenplay drafts, showcasing the versatility of AI in creative professions.
Looking ahead, emerging trends in AI include advancements in natural language processing (NLP), which could lead to more sophisticated virtual assistants and improved human-computer interactions. The development of explainable AI (XAI) is another critical area, focusing on creating AI systems whose actions and decisions are transparent and understandable to human users.
However, the increasing integration of AI into society raises important ethical considerations and challenges. Concerns about data privacy, algorithmic biases, and the potential displacement of jobs due to automation need to be addressed. It is crucial for stakeholders, including policymakers, businesses, and the public, to collaborate on establishing regulatory frameworks that ensure the responsible and equitable development of AI technologies.